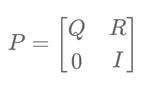
Imagine playing a board game where you move between different states based on dice rolls. Each space on the board represents a state, and you transition between states based on the dice outcome – this is the basic idea of a "Markov chain."
Now, imagine some spaces on the board are special rooms with no way out once you enter them. These special rooms are called "absorbing states." Once you step into one, you're stuck there indefinitely.
In the context of the dice game, the "absorbing Markov chain" is a version of the game where certain spaces act like traps.
Imagine you're analyzing a mix of stocks in your investment portfolio. The stocks are divided into three categories: "Tech," "Energy," and "Consumer Goods." The movements between these categories depend on market trends and other financial factors.
Now, let's assume that stocks in "Tech" and "Energy" can transition between each other based on market conditions. But stocks categorized as "Consumer Goods" have an interesting trait – when they perform well, they tend to remain in that state due to steady consumer demand.
State 1: "Tech" stocks
State 2: "Energy" stocks
State 3: "Consumer Goods" stocks
"Consumer Goods" stocks are the absorbing state here. Once stocks end up in this state, they usually stick around for a significant period due to consumer behavior. It's like a trend that maintains itself.
The absorbing Markov chain helps you understand the probabilities of stocks transitioning between these states and eventually landing in the absorbing state of "Consumer Goods." Once they're there, you know they'll likely stay for some time due to the absorbing property.
In an absorbing Markov chain, the numbers linked to the edges of the graph represent transition probabilities. These probabilities indicate how likely it is for the system to move from one state (node) to another state (node).
('Tech', 'Energy'): 0.4. This line signifies a transition probability of 40% from state 'Tech' to state 'Energy'.
('Energy', 'Tech'): 0.3. In this case, the probability of transitioning from 'Energy' to 'Tech' is a 30% chance of making this transition.
('Tech', 'Consumer Goods'): 0.2. This line represents a transition probability of 20% from 'Tech' to the absorbing state 'Consumer Goods'.
('Energy', 'Consumer Goods'): 0.1. Similarly, the probability of transitioning from 'Energy' to 'Consumer Goods' is 10%.
The transition probabilities for an absorbing Markov chain are typically represented in matrix form:
$$P = \begin{bmatrix} 0.4 & 0.2 & 0.4 \\ 0.3 & 0.3 & 0.4 \\ 0 & 0 & 1 \end{bmatrix}$$
Here, each row represents the current state, and each column represents the next state. For example, the probability of transitioning from "Tech" to "Energy" is 0.4, as seen in the first row, second column of the matrix.
Absorbing Markov chains are analyzed using the fundamental matrix. For an absorbing Markov chain, the transition matrix can be partitioned as follows:
$$P = \begin{bmatrix} Q & R \\ 0 & I \end{bmatrix}$$
Here:
- Q: Transition probabilities among transient states
- R: Transition probabilities from transient states to absorbing states
- 0: Zero matrix
- I: Identity matrix for absorbing states
The fundamental matrix is given by:
$$N = (I - Q)^{-1}$$
This matrix helps calculate the expected number of steps before being absorbed into an absorbing state. For example, if you start in "Tech," you can use the fundamental matrix to determine the average number of transitions required to reach "Consumer Goods."
The probabilities of being absorbed into each absorbing state are given by:
$$B = NR$$
This matrix indicates the likelihood of eventually ending up in each absorbing state from any given transient state.
By applying these calculations, you can analyze how a portfolio of stocks transitions over time and determine the long-term behavior of the system.
Écrire commentaire